TALK DETAILS
Behavioral Classification of Sequential Neural Activity Using Time Varying Recurrent Neural Networks 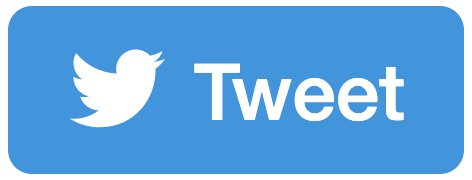
Yongxu Zhang β Shreya Saxena
28 September 2022
Classification of behavior from multi-regional neural data sequentially in time can help in the early detection of behavior, which may provide important ways to provide corrective neural stimulation before the onset of behavior. Recurrent Neural Networks (RNNs) are designed for time-series data: they take in sequential inputs and predict the class of the sequence using recurrent hidden states that are able to retain a memory of previous inputs. However, standard RNNs cannot guarantee the classification at earlier points of the sequence; they are traditionally designed to achieve correct classification at the end of the sequence. To help the network utilize all temporal features of the input and to enhance the memory of an RNN, we propose a novel approach: RNNs with time-varying weights, here termed Time-Varying RNNs (TV-RNNs). These models are able to not only predict the class of the sequence correctly but also lead to accurate classification earlier in the sequence than standard RNNs. In this work, we focus on early sequential classification of brain-wide neural activity across time by using TV-RNNs as subjects perform a motor task. We show the utility of our method on simulated data, and show its performance on two different experimental datasets: (a) widefield calcium imaging that records the activity across the dorsal cortex while mice perform a βlever pullβ task, and (b) brain-wide fMRI data recorded while subjects perform a grip force task. When trained to perform binary classification of behavior, we find that TV-RNNs outperform standard RNNs in early classification of the sequence. To understand the model mechanisms, we visualize the time-varying weights which show that the change of the TV-RNNs weights is larger at the end of the sequence than at the early timepoints in the sequence. We also compute the SHAP value that indicates the contribution of different brain regions to the classification, and find that somatosensory and motor areas several seconds before the βlever pullβ are more important in behavioral decoding, and the PreSMA region contributes more to the grip force task. Thus, we show that (a) TV-RNNs outperform standard RNNs, (b) we are able to understand the classification mechanisms of TV-RNNs, (c) we are able to accurately pinpoint the effect of different regions on behavioral decoding.
doi.org/10.57736/nmc-f346-d239π
VIDEO RECORDING
QR CODE
