TALK DETAILS
Brain-like visual surround suppression in generic CNNs: successes and limitations 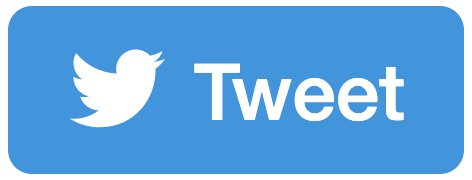
Annie DeForge — Xu Pan, Odelia Schwartz
28 September 2022
Spatial context plays an important role in visual processing and perception. A rich set of surround effects that involve nonlinear interactions between the center and surround have been found in Primary Visual Cortex (V1). A general summary is that surround suppression is prevalent and the amount of suppression depends on the visual similarity between the center and surround. In recent years, convolutional neural networks (CNNs) have successfully modeled neural behavior across the visual cortices. However, there have been limited studies addressing the multitude of contextual effects that have been documented experimentally. In this study, we did in-silico simulations on CNN neurons in Alexnet and VGG16 following the experimental neurophysiology paradigms as much as possible. We found that a significant amount of CNN neurons, especially in middle layers, behave similarly to cortical neurons regarding the surround suppression effects, including: surround suppression is strongest when the surround has the same grating orientation as the center regardless of whether the center orientation is optimal or not; the suppression difference between the preferred surround and orthogonal surround is significant only when the center contrast is high; and spectrally matched noise induces more suppression than naturalistic images in line with V2 data. We also found some mismatches between the CNN and cortical neurons: the geometric effect of surround suppression for collinear versus parallel surround stimuli is less pronounced in CNN neurons, and the contrast effect on the peak of the diameter tuning curve is seen in some neurons but does not reach statistical significance in most layers. The unexpected alignment between the CNN and cortical neurons regarding some surround effects extends the ability of the CNN as a model of visual cortices. The mismatches we found could inspire future CNN designs to address them, including the role of divisive normalization, lateral connections and feedback.
doi.org/10.57736/nmc-f847-38bd📋
VIDEO RECORDING
QR CODE
