TALK DETAILS
Exploring the Mouse Visual Cortex 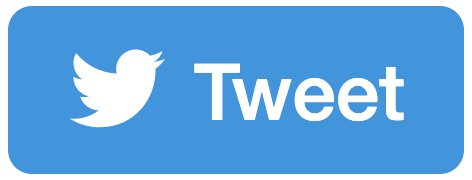
Ananna Biswas — Abraham George, Asutosh Routa, Bhavika Gopalani, Cristian Lazo Quispe, Jongwon Yun, Joshua Philippe Olorocisimo, Manasa Boggarapu, Rouhong Wang, Tanushka Bhatia
28 September 2022
In this study, we aim to broaden our understanding of the mouse’s primary visual cortex by modeling data provided by the datasets from Stringer et al. (2019) and the Allen Institute. We investigated the roles of the different excitatory and inhibitory cell types in the visual cortex whose functions remain unclear.
Using the Stringer dataset, we explored the correlation between the activity of excitatory cells in the mouse visual cortex and mouse running behavior without any visual input. Principle Component Analysis (PCA) was applied to reduce the dimension of our neuron activity data of 11983 excitatory neurons into 2000 principal components that contain 88.82% of the cumulative explained variance. Then, we modeled the relationship between neural activity data and running speed data using a linear regression model and achieved an RMSE of 1.86 and 4.21 cm/s on the training and testing dataset, respectively. Moreover, we have found out that there is a positive correlation between running speed and each layer of neurons in the visual cortex. Finally, our preliminary data supported the possibility of predicting running speed from excitatory neural activity in the visual cortex.
Through the Allen Dataset, we were able to go a step further and gather more insights into the inhibitory neurons, specifically the vasoactive intestinal peptide (VIP) and somatostatin (SST) neurons. To elucidate their functions, we built an image identity decoder from the activity of hundreds of neurons from each cellular subtype. We plotted the activity of each cell in multi-dimensional space and used dimensionality reduction techniques such as PCA and t-SNE. Then, we built a decoder using an SVM algorithm. We found that SST cells provided better clustering and decoding (61.46% accuracy) of images compared to VIP cells (31.13% accuracy). Interestingly, VIP cells had a higher response to image omissions. This may be because VIP cells are known to enhance responses to weak stimuli, while SST cells respond more to strong stimuli. Thus, we were able to show that SST cells have a highly tuned response to image identification, while VIP cells play a larger role during image omission.
Together, our study shows that excitatory cells in the visual cortex may have an added role in navigation aside from processing visual input. In addition, inhibitory cell subtypes show differential roles in visual processing. We have provided separate insights into the roles of different cell types in the visual cortex, but how they interact together in visual and non-visual tasks merits further investigation.
doi.org/10.57736/nmc-7a64-6a3a📋
VIDEO RECORDING
QR CODE
