TALK DETAILS
Mooney Face Image Processing in Deep Convolutional Neural Networks Compared to Humans 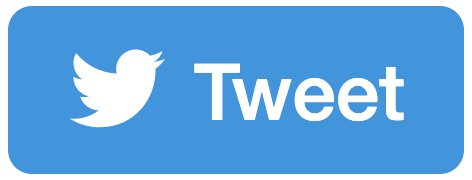
Astrid Zeman — Tim Leers, Hans Op de Beeck
28 September 2022
Deep Convolutional Neural Networks (CNNs) are criticised for their reliance on local shape features and texture rather than global shape. We test whether CNNs are able to process global shape information in the absence of local shape cues and texture by testing their performance on Mooney stimuli, which are face images thresholded to binary values. More specifically, we assess whether CNNs classify these abstract stimuli as face-like, and whether they exhibit the face inversion effect (FIE), where upright stimuli are classified positively at a higher rate compared to inverted. We tested a CNN trained for facial recognition (DeepFace) and found that the network performs perceptual completion and exhibits the FIE, which is present over all levels of specificity. By matching the false positive rate of CNNs to humans, we found that the network performed closer to the human average (85.73% for upright, 57.25% for inverted) for both conditions (62.70% for upright, 42.26% for inverted). Rank order correlation between DeepFace and humans across individual stimuli shows a small but significant correlation in upright and inverted conditions, indicating a relationship in image difficulty between observers and the model. We conclude that despite texture and local shape bias of CNNs, which makes their performance distinct from humans, they are still able to process object images holistically.
doi.org/10.57736/nmc-d07a-e915📋
VIDEO RECORDING
QR CODE
